
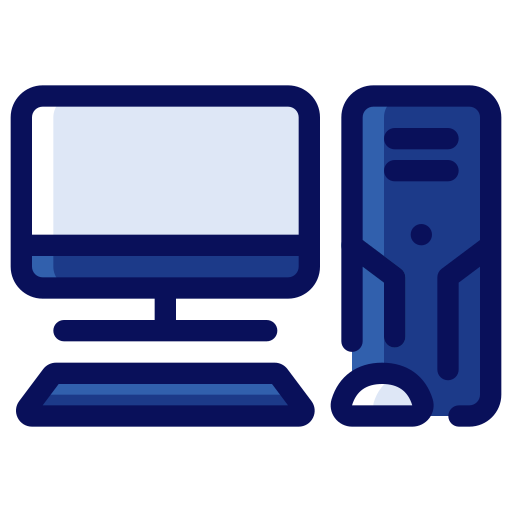
play on bad private servers i guess
Oh damn, I ate too many selfhosted letters, I’m afraid I’ll have to vomit.
Vanilla vmangos
TBC cmangos
WotLK AzerothCore TrinityCore
Haaaa, I think it’s finally over…
SinglePlayerProject
Urgh. Sorry. Nothing to see here, move along everybody!
Well during that time you will have lost quite a lot of skin cells, so in a manner of speaking you literally have some skin in this game. Around 328 grams going by an average loss of 3.6kg/year I took from some random website.